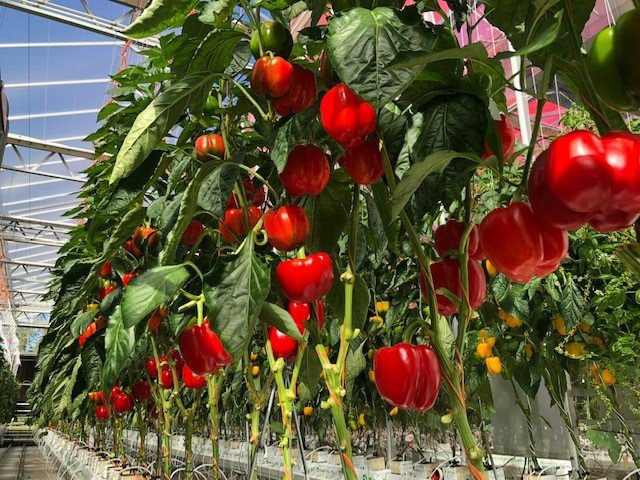
PROGRAM 2 -
Advanced crop health and yield
Program 2 Lead,
Professor Ulrike Mathesius
In Program 2, we’re aiming to develop and enhance strategies for minimising crop stress and disease through early detection and improved microbiomes.
We’ll be working on development, targeted refinement and advanced capabilities in robotics and machine learning, and AI will accelerate opportunities and outcomes for the PC industry in several ways: efficiency, resource optimisation, early detection and control, yield consistency, environmental sustainability, and labour efficiency and safety.
Our students and researchers will be trained in the use and integration (with POs) of advanced data science, machine learning, and AI techniques, as well as cutting-edge robotics and automation. They will also gain skills in predictive and intervention tools for important crop pests and diseases.
Program 2 Projects
Project 2.1 - Artificial intelligence to monitor crop health and yield
Chief Investigators: Mathesius, Guo, Singh, Elgidi, Ghannoum, Sharwood, Riegler, Tjoelker
Partners : Vertical Patch, BizData, Intersect, APPN
In recent years, there has been a shift toward adopting advanced technologies (e.g. remote sensing, drones, satellite imagery) to monitor crop conditions.
However, the vast amount of data generated by these technologies presents a challenge in terms of data analysis and interpretation, necessitating the integration of advanced data science, machine learning, and AI techniques.
These advanced techniques can not only enhance crop management precision but also empower growers to make informed decisions, leading to improved crop yields and resource efficiency in controlled greenhouse environments.
Program 2’s students and researchers will be trained in…
2.1.1. Data generation and collection
Precision Sensor Integration: Controlled greenhouses are equipped with an array of sensors to monitor environmental conditions, such as temperature, humidity, CO2 levels, nutrient concentrations, and crop health. Machine learning models can leverage the continuously produced data from these sensors in real-time to analyse conditions and make recommendations.
Robotics for Data Collection: Robotic platforms can be deployed for data collection, and performing tasks like imaging, pollinating, disease control, harvesting, etc. The robots can navigate through the greenhouse autonomously, enhancing data accuracy and enabling more comprehensive monitoring of crop conditions.
2.1.2. Growth Optimisation.
Computer Vision for Crop Monitoring: Multimodal (spectral and structural) imagery captures detailed images of plants in their growth cycle. Computer vision techniques will be applied to extract growth indicators and identify signs of stress, disease, or pest infestations, providing necessary data and statistics for growth and health.
Climate Control: Machine learning algorithms can continuously analyse environmental data and predict optimal climate conditions for specific crops. By adjusting factors, like temperature, humidity, and light intensity in real-time, the system can ensure that plants thrive and grow to their maximum potential, leading to higher yields.
Nutrient Management: AI systems can monitor nutrient levels in the soil or hydroponic solutions and adjust nutrient delivery based on crop growth stage and matched requirements. This level of precision supports optimal plant development, while minimising nutrient wastage.
2.1.3. Yield Prediction.
Historical Data Analysis: Machine learning can process historical data from greenhouse operations, such as previous crop yields, environmental conditions, and management practices. By identifying patterns and relationships within this data, AI models can make highly accurate predictions of future crop yields. This information assists growers to effectively and efficiently plan production and supply chain activities.
Crop Health and Disease Prediction: Predictive models can be trained to anticipate the onset of diseases or pest infestations based on environmental conditions and plant health data. Early identification of potential threats enables growers to take preventive measures, such as targeted treatment, to protect their crops, minimising loss and supporting consistent yield.
Project 2.2 - Robotics for Crop Monitoring and Management
Chief Investigator: Guo
Partner Investigator: Troughton
Industry Partner: XAG Australia
The use of robots, including drones/UAVs (unmanned aerial vehicles), remains in its infancy in the PC sector. Unmanned ground vehicles (UGVs) suitable for horticulture are still far from commercialisation, although many intermediate results have been incorporated into agricultural equipment—from harvesting to precise herbicide application.
Partner Organisation XAG Australia will provide access to a UAV model for researcher training, and for research into the development of newer versions that are more suitable for glasshouses and polytunnels.
The Centre will further leverage XAG’s expertise in robotics and the Internet of Things (IoT) for sensing and building IoTs for the various facilities to streamline data acquisition and transmission.
Project 2.3- Integrating Microbiomes for Sustainable and Profitable Protected Cropping
Chief Investigator: Singh and Elgidi
Partner Investigators: Ford, Potter, O’Hara
Standard practices in PC favour the survival and dispersal of problematic crop pathogens. For instance, certain practices, like substrate handling and movement can increase pathogen survival, while irrigation systems and movement of equipment can disperse pathogens within and between growing facilities, resulting in costly mitigation approaches, increased use of pesticides and fertilisers, and economic losses.
This project will work in partnership with key stakeholders in PC, including growers, to provide an effective tool for Integrated Disease Management (IDM). Specifically, this will combine genomics, microbiome analysis, conventional disease surveys, and AI to predict disease incidence and severity. It will support the development and improvement of microbial (inoculants, syncoms), metabolite (originated from plant and microbes), and management intervention tools to minimise the impacts of disease on crop productivity and quality.
New products and knowledge will be commercialised by participating industries, and relevant training will be provided for industrial stakeholders to ensure wider adoption for successful commercial outcomes. The training framework for this project will include research, leadership, and entrepreneurial skills developed and employed in industrial settings.
The Benefits
Prevention of Economic Losses
Novel strategies and tools will be developed to help prevent economic losses caused by crop failure, pests and diseases, ensuring sustained productivity and profitability in PC environments.
Reduced Environmental Impact
Using the IDM approach can reduce chemical inputs, lower management costs, boost profitability, and mitigate potential harm to non-target organisms and the environment from some chemical inputs.
Resilience to Climate Change
Microbiome management can enhance crop resilience to disease pressure in PC environments, crucial in a changing climate with extreme weather events and shifting disease patterns.
Integrating machine learning and AI technologies into protected cropping has the potential to revolutionise the way we monitor, manage, and optimise crop growth, health, and yield.
These technologies can empower growers to achieve higher efficiency, lower resource consumption, and increased crop yields, while ensuring a more sustainable and controlled agricultural environment.